CVPR2020にリモート参加した。時差があるので夜中の1:00に始まるので大変だった。CVPR自体は3日だが、前後にWorkshopが付いており、合計6日参加した。
費用は非会員なので$350だった。安い。知の交流を守っておられる運営陣の方々に敬意を表したい。
Table of Contents
Sunday: LID Workshop(Learning from Imperfect Data)
Learning from Imperfect Dataとは
画像/動画処理の分野ではタスクが高度化している。
それにより、Deep Learningに必要な教師データ作成作業(アノテーション)のコストは上がり続けていた。その結果、完全な教師データを人手で作成することは不可能になった。DataがImperfectであるという制約は現実問題、常に受け入れざるを得ない。
そのため、Imperfect Dataを使って学習するアルゴリズムを開発する必要がある。
問題は、ほとんどの重要なケースがImperfect(欠落)部分に含まれることである。これを象徴的に理解するために、「横転したトラック」の例を用いる。
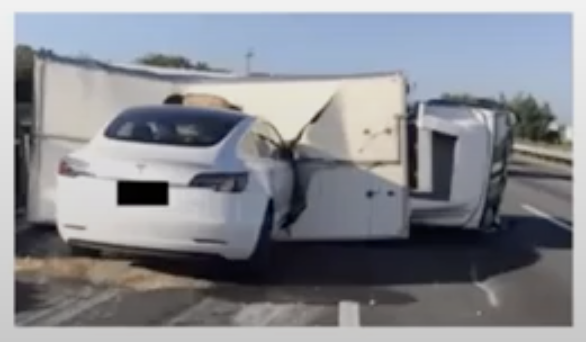
これはコーナーケースであり、完全な教師データを作ることは起こりうるすべての事故を列挙することであり、原理的に不可能である。
問題は、再掲ではあるが、「すべてがコーナーケースである」ことだ。それを受け入れなくてはならない。
LIDは今年で2回目の開催だ。「古参なんですよ〜」と将来言っていきたい。
不完全性の種類と対策
LIDに対するアプローチには以下が知られている。
1) 弱教師学習
- 弱教師の例1: 画像に何が写っているかのラベル。猫が写っていることは知らされるが輪郭は不明(Imperfect)。
- この手法はBest Paper Awardの3D Reconstructionの論文でも採用。
- 弱教師の例2: 各Instanceについて重心の点のみが与えられる。輪郭は不明(Imperfect)。
- 画像間の類似性を用いてpseudo(シュード)教師データ生成を行う。
- contractive lossなどもこれに該当。
2) 教師なし学習
- 教師なし学習の例1: featureを学習
- 教師なし学習の例2: Neural Architectureを学習
3) few shot / 0 shot学習
これは教師あり学習ではあるが、いくつかのクラスにおいては、教師データが2~3枚であったり0枚であるという場合である。imbalanced問題ともいう。
- 例えば上記の例「横転したトラック」のようなコーナーケースをうまく分類できるかというのがこれに該当する。
教師データがなくても、「正しく」特徴が学習されていれば、上記を予測できる可能性はありうる、ということに基づいている。
list of invited talks:
1 2 3 4 5 6 7 |
8:40-9:10 Professor at Johns Hopkins University Alan Yuille Invited talk 1: You Only Annotate Once, or Never 9:10-9:40 CEO at Ariel AI Inc. / Associate Professor UCL Iasonas Kokkinos Invited talk 2: Learning 3D object models from 2D images. 9:50-10:20 Director of Research at Mapillary Peter Kontschieder Invited talk 3: Computer Vision with Less Supervision 10:30-11:00 Research Scientist at Google Boqing Gong Invited talk 4: Towards Visual Recognition in the Wild: Long-Tailed Sources and Open Compound Targets 11:10-11:40 Staff Research Scientist at Facebook Research Zhicheng Yan Invited talk 5: Decoupling Representation and Classifier for Long-Tailed Recognition 14:00-14:30 Professor at University of California, Merced Ming-Hsuan Yang Invited talk 6: Show, Match and Segment: Joint Weakly Supervised Learning of Semantic Matching and Object Co-segmentation 17:50-18:20 Professor at University of Adelaide Chunhua Shen Invited talk 7: Single shot instance segmentation |
talk1: You Only Annotate Once, or Never
Alan Yuilleの哲学によれば、人間とくに幼児はLIDを行なっている。確かに、我々はすべての事故を経験していないにも関わらず、事故の画像を正しく判定できる。
- optical flow(動体分離を含む)
- stereo correspondence(目が二つあるのでその整合的な処理)
- depth / 3D reconstruction
- この見地からは、Active Interactionも重視される。
主張1=「We should train with very little annotated data.」
主張2=「We should allow “worst enemy” to test rather than random data.」
https://paperswithquery.com/paper/1911.11230
本題と関係ない(?)ところでUnNASが面白い。
https://paperswithquery.com/paper/2003.12056
talk2: Ariel AI
人間の再構成
Mapillary(スウェーデン企業)はPlanet Scale Datasetを自動生成する話
wikipediaによると、10億枚を突破したらしい。
https://en.wikipedia.org/wiki/Mapillary
BoqingはDomain Adaptationの話
ZhichengもLongtailの話だが、Decouplingが主題
Show, Match and Segmentはconsistencyを入れまくってマッチングとco-segmentationをjoint trainingする話
BlendMaskは、Blender機構を持ったセグメンテーション。YOLACTよりよい。
https://paperswithquery.com/paper/2001.00309
https://github.com/aim-uofa/AdelaiDet
https://paperswithquery.com/paper/2003.05664
https://paperswithquery.com/paper/1912.04488
CondInstは、Instance Segmentation。
難度はInstance Segmentation >> Semantic Segmentaion。例えば人々(Semantic)の中にいる人を個別に(Instance)見つけなければいけない。
FCNではInstance Segmentationができない。(InstanceとInstanceが接している場合、区別できない)
https://paperswithquery.com/paper/1605.06211
CondInstはこのInstance個数分のmask headを持っている。
SOLOは位置情報を使ったセグメンテーション。contrastive learning
workshop challenges:
Track1: Weakly Supervised Segmentation
画像のセグメンテーションを行う。ただし「画像全体に対して割り当てられたラベルのみ」を用いて。
通常はpixelwiseの教師データが与えられるので、高難度のタスクである。
- Class Activation Map AutoGeneration
この方法では、pseudoラベルをCNNの途中出力から作成するが、それだけでは足りないので、cross image relationshipsを取り出す方法が鍵となる。
- IRNet 2019 SOTA
Track2: Pointly supervised Scene Parsing with Uncertainty Mixture
- 上記の通り、各インスタンスの重心点のみが与えられるので、自然なモデリングはMixtureモデルになる。
- 今回の成功者はGamma Mixture
- EM Algorithm
Track3: Weakly Supervised Object Localization
Monday: From SIFT to Differentiables
完全に実務とは関係ない趣味の理論的探求として、今回はこれを選択した。開設されたアルゴリズムは以下の通り。
- SIFT
- Hessian Laplace
- Harris
- SURF
- Edge Foci
- MSER
- FAST
- GLOH
- CHoG
- DAISY
- LIOP
- LUCID
- ASIFT
- DSP-SIFT
- Hashing-SIFT
- BRIEF
- PCA-SIFT
- Convex Optimization
- Exemplar CNN(転換点)
- Bischof
これはDeepCompareとほぼ同じだが、精度が悪く、タイミングも2008年と悪かった(DeepLearning以前)。引用数が21にとどまっている。 - DeepCompare
Siameseネットワークに似た発想。 - TFeat(2016, link_to_paper_british_machine_vision_association)
Triplet(x,y,z)を使い、yがxとzのどちらに似ていると判定されるかが学習率の符号に反映される。 - L2-Net(2017, link_to_paper_CVPR)
- Binary L2-Net
- HardNet
- SOSNet(2019, CVPR)
- LIFT
- LF-Net
- Learning to find good correspondences
- SuperPoint
- IMIPs
- DELF
- D2Net
- UR2KiD
- SuperGlue
- DeMoN
- PoseNet
- Local Scene Coordinates
- AffNet
解説されてないがベンチマーク上存在が確認されているアルゴリズム
- √SIFT
- AKAZE(おなじみの)
- ORB
- FREAK
- HessianAffineNet-SIFT
- GeoDesc
- ContextDesc
- LogPolarDesc
- R2D2(D2Netの流れ)
Korniaというライブラリについて:
https://github.com/kornia/kornia
一言で言うと、torch+GPUで(Traditional) Computer Visionのアルゴリズムを動かせるライブラリだと思う。速そうだった。
Tuesday+Wednesday+Thursday: Main Tracks(3days)
Opening
初のリモート開催であることの報告。
Award受賞者の教授は、CVの権威。
Satya Nadella Firechat
いくつかMicrosoftの注力分野とSatyaのビジョンが紹介された。
- 工場で作業をする人を上から撮ったときに、ほぼ全ての物体種類と行動種類が検知されているデモ。
- クロマキー合成を、特殊背景なしに行うデモ。Zoomのバーチャル背景とほとんど同じ。
- HoloLenseのデモで、自分の等身大ホログラムが、音声翻訳を利用して異国語で話をすると言うデモ。
- (めっちゃ多いので後で書く)
そして学会が始まったが、今回は、のっけから504。twitterも荒れていた。しかしながら運営の努力により20分で復旧した。
1) CvxNet: Learnable Convex Decomposition
立体の学習だが、Convex(凸体)が学習しやすいことに着目し、任意の物体をConvexに分解した上で、学習することを目指す。着想が鮮やかでHintonっぽい。Hintonグループ。
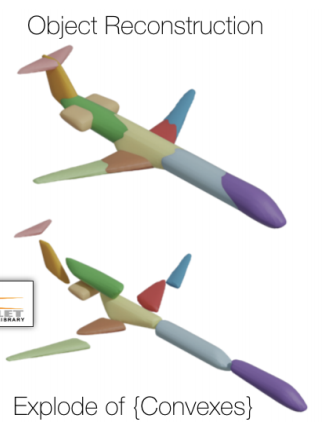
2) PIFuHD: Multi-Level Pixel-Aligned Implicit Function for High-Resolution 3D Human Digitization
Shunsuke Saito氏のPIFu(パイフ)。服のきめ細かさまで再現できるHD版。
3) Unsupervised Learning of Probably Symmetric Deformable 3D Objects From Images in the Wild
→これはすごいね。AutoEncoderでView/Depth/Textureに分解して再構成(再構成は難しいのでここにトリックあり)で、FineTuningなしで驚愕の精度。
4) Peek-a-Boo: Occlusion Reasoning in Indoor Scenes With Plane Representations
5) FastDVDnet: Towards Real-Time Deep Video Denoising Without Flow Estimation
超高速に動画のノイズ除去を行う。
6) Seeing the World in a Bag of Chips
反射のみを分離して、kinectで得たDepthデータから反射の中に映り込んだ情報の再構成を行う。
Inter-Reflectionのシミュレーションもできる。
7) Mapillary Street-Level Sequences: A Dataset for Lifelong Place Recognition
写真によって地図を検索するための学習用データセットを提供する。
8) Image Based Virtual Try-On Network From Unpaired Data
- Virtual try-on(試着)
- DensePose/PSPNetなど既存のネットワークも使っている
9) PSGAN: Pose and Expression Robust Spatial-Aware GAN for Customizable Makeup Transfer
10) Disentangled Image Generation Through Structured Noise Injection
11) D3Feat: Joint Learning of Dense Detection and Description of 3D Local Features
12) Circle Loss: A Unified Perspective of Pair Similarity Optimization
13) Semantic Pyramid for Image Generation
14) Contextual Residual Aggregation for Ultra High-Resolution Image Inpainting
15) Upgrading Optical Flow to 3D Scene Flow Through Optical Expansion
16) BlendMask: Top-Down Meets Bottom-Up for Instance Segmentation
17) Deep Polarization Cues for Transparent Object Segmentation
18) Approximating shapes in images with low-complexity polygons
19) Robust 3D Self-Portraits in Seconds
- 3D Scanを、Bundle Adjustmentを使ってPIFUの予測にAlignする、PIFusion(パイフュージョン)というアルゴリズム。
- 地道と感じた。
20) Computing the Testing Error Without a Testing Set
21) Learning Interactions and Relationships Between Movie Characters
22) Understanding Human Hands in Contact at Internet Scale
23) You2Me: Inferring Body Pose in Egocentric Video via First and Second Person Interactions
24) Telling Left From Right: Learning Spatial Correspondence of Sight and Sound
videoを入力として学習して、Monaural音声をStereo音声に変換するDNNを作る。
25) Learning Temporal Co-Attention Models for Unsupervised Video Action Localization
26) Deep Relational Reasoning Graph Network for Arbitrary Shape Text Detection
27) Sketch Less for More: On-the-Fly Fine-Grained Sketch-Based Image Retrieval
28) BBN: Bilateral-Branch Network With Cumulative Learning for Long-Tailed Visual Recognition
29) ABCNet: Real-Time Scene Text Spotting With Adaptive Bezier-Curve Network
30) Learning User Representations for Open Vocabulary Image Hashtag Prediction
31) Few-Shot Pill Recognition
32) 3DSSD: Point-Based 3D Single Stage Object Detector
33) SESS: Self-Ensembling Semi-Supervised 3D Object Detection
Friday: 2nd Workshop on Learning from Unlabeled Videos (LUV)
Imperfect DataとUnlabeled Videoの違い